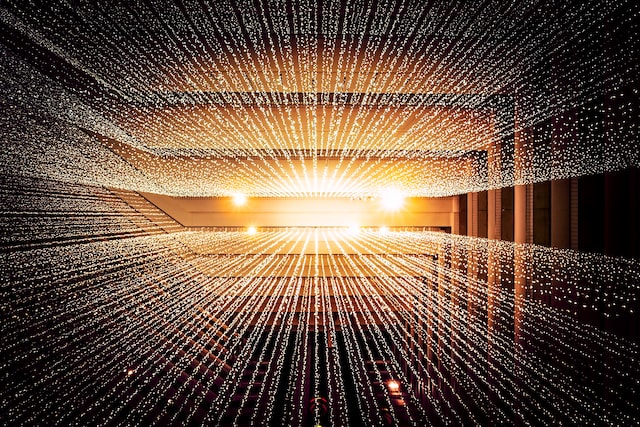
Science Journalism is like a blurry image of science. Image courtesy of Joshua Sortino
I really enjoyed the recent New Yorker article by Ted Chiang 1 that draws an analogy between the way that lossy photo formats like JPEG store representations of images and the way that large language models store knowledge. I found this analogy to very useful. Its a great way to describe the current state of these models to folks tangential to ML and NLP without dropping into transformer architecture and attention mechanisms. The post has also drawn some criticism2 from scientists working in deep learning for not being “in keeping with our scientific understanding of LMs or deep learning”. Whilst Chiang may miss the finer strokes, the picture he paints is broadly representative. In a very meta way, his own work is like a blurry JPEG of how LLMs work. You might even consider that scientific journalism in general is like a blurry JPEG of scientific writing. I believe that in this context, such broad metaphor is ok most of the time. Let me explain.
Scientific Journalism vs Scientific Writing
Scientific Journalism and Scientific Writing have two different but complementary purposes. Scientific papers are set up to be precise and full of specific details that describe exactly what was done and what the results were and give other scientists a sort of “recipe” for recreating the work. They need to be uber-specific and highly technical and usually end up landing somewhere between “a bit dry” or “undecipherable” for average non-specialist readers.
The intended readers of scientific papers are the group of the authors’ peers who may want to reproduce or build upon their work. However, scientific news articles are supposed to appeal to a much broader lay-audience. For scientific journalists, education of the audience is a given, it’s ’table stakes’ for partaking in this particular corner of the attention marketplace. However, journalists are ultimately incentivized by sales of news products, be it ye-olde physical newspaper or online subscriptions. Therefore, they make their articles appealing to a broad audience by offering entertainment and intrigue. It’s not possible to clinically and precisely convey information about highly technical scientific work whilst also entertaining a broad audience. Some precision must be sacrificed, some concepts simplified. A study on good scientific news reporting by Louis and Nenkova found that high quality articles will often use creative language and metaphor to make complex effects relatable 3. By making science accessible and entertaining, we can “[raise] awareness and [convince] the public that a scientific issue needs more political, financial and intellectual attention.” 4.
I should also briefly touch on length. Scientific papers are typically around 5000-7000 words in length 5 whereas news articles are typically 600-700 words in length depending on the area that they cover 6. Let’s face it, even if your editor lets you have more than a couple of sides in the paper or magazine, if you write for much longer than that, you’re probably going to lose your audience. Therefore, we need about a 90% information compression rate to get from scientific paper to news article. Scientific journalists are doing a very tricky job as they try to balance all of these constraints.
Is It Ok That Journalists Use Clumsy Metaphors to Describe Precise Concepts?
This is context dependent but, most of the time, yes.
According to the theory of cognitive constructivism, popularised by psychologist Jean Piaget 7, we learn by incrementally adding to and building on top of our experiences. Slowly and methodically deepening our understanding of the world. Consider how education works for young children. When your 3 year old asks “why is the sky blue?” we typically start with “because of science” and probably don’t tell them about Rayleigh scattering effect which causes electromagnetic radiation to scatter off of strongly-bound charged particles 8. We teach simplified models of complex processes to provide frameworks to hang concepts from and then once enough information has been accumulated, we re-visit those simplified models and flesh them out. In elementary physics we might teach that atoms are the smallest quanta of matter and later in advanced physics, we refine this model: atoms are a thing but, we also have sub-atomic particles like quarks and gluons. As an adult we continue these incremental learning processes. We might learn simple rules of thumb that help us with our jobs and our personal lives but as we acquire more experience and make more mistakes we’re able to finesse these rules into more complex models and foresee edge cases.
If we want people to engage with modern science, we need to make it accessible and to make it accessible we need to provide simplified and relatable models of what is happening ‘behind the curtain’. This process is akin to ‘good enough’ lossy compression. Once people are engaged, then the curtains can be drawn open.
When Is It Not Ok To Use Clumsy Metaphors To Describe Precise Concepts?
During ethical and political debate or generally in high-stakes decision-making contexts. This is when simplified arguments run the risk of becoming strawman arguments 9 because they do not take into consideration the nuanced “real” nature of a situation. This in itself is a bit of a nuanced position and a difficult one to practice. After all, in the modern political and legal landscape, the people who make important decisions are often not expects in the field that they are making decisions about. Such individuals often need to rely on crutches and metaphors as part of their process (and perhaps therein lies another problem). In these situations it’s important that nuances are called out and well represented to ensure healthy debate.
Continuing with our theme, you might enjoy looking at a slightly pixelated photo that your Grandma on her 15-year-old camera phone during her vacation but you would definitely refrain from convicting someone of a crime if the evidence is a blurry photo in which you don’t see their face.
Objections to LLMs and the “Lossy Photos” Analogy
So why might it be problematic to think about LLMs as a lossy photo of the web?
Well, obviously the blurry photo analogy is a simplification and it doesn’t really do justice to the cool stuff these models are capable of. We know that deep learning model performance improves when models are exposed to different types of tasks 10 11 and different types of data (e.g. both images and text 12) so a large model that’s been exposed to lots of different data types (like a whole great big bunch of internet content circa 2021) should, in theory, be very capable at generalising to new tasks rather than just memorising stuff. As my good friend and fellow NLP specialist Dan Duma 13 commented, Chiang’s point that ChatGPT can’t do maths kind of side-steps the fact that it has learned “the math” of argument and storytelling and iambic pentameter 14.
Indeed, in practice, ChatGPT sometimes plagiarises things but more often than not, it’s capable of generating novel outputs and completing “classical” NLP tasks like classification and named entity recognition with zero fine tuning. However, I think if we’re less literal about the memorisation thing, the metaphor still stands. Sure, ChatGPT has literally rote learned some stuff, but it’s also learned some “lossy” rules of thumb. Some of them are helpful, like, producing boilerplate for a formal letter or a piece of code. Some, clumsily hidden behind OpenAI’s content policy system, are harmful, such as the links between genders and certain professions that the system has internalised 15.
So yes, the lossy photos analogy doesn’t quite fit once you get into the detail but I think it’s a great mental crutch for folks who aren’t used to LLMs and who are probably expecting a crisp but much lower resolution, fact-checked experience like that of systems like Siri or Alexa. However, once you start really thinking about LLMs, it’s important to realise that things are more nuanced and that these kinds of systems can be are pretty smart in some situations. As Sam Bowman suggests 16, perhaps by under-stating the capabilities and impact of these AI systems, there’s a danger that we turn people off to the very real social and political challenges that they introduce which we are already seeing emerge today 17.
Conclusion
Scientific journalism is a process that applies “lossy compression” to precise scientific literature in order to make it more immediately accessible to a wider audience. The metaphors and analogies produced as part of this process provide mental frameworks upon which non-technical readers can build. Most of the time, for most people, this lossy compression paints a “good enough” picture, making science appealing and accessible to a wide audience and building socio-political support and engagement with scientific work. However, it’s right to object to these simplified, compressed views of scientific work when engaged in debates concerning the ethical, societal and political nuance surrounding them.
-
Chiang, T. (2023, February 9). ChatGPT Is a Blurry JPEG of the Web. The New Yorker. https://www.newyorker.com/tech/annals-of-technology/chatgpt-is-a-blurry-jpeg-of-the-web ↩︎
-
Andrew Lampinen on Twitter: “Ted Chiang is a great writer, but this is not a great take and I’m disappointed to see it getting heavily praised. It’s not in keeping with our scientific understanding of LMs or deep learning more generally. Thread: 1/n” https://twitter.com/AndrewLampinen/status/1624422478045913090 ↩︎
-
Louis, A., & Nenkova, A. (2013). What Makes Writing Great? First Experiments on Article Quality Prediction in the Science Journalism Domain. Transactions of the Association for Computational Linguistics, 1, 341–352. https://doi.org/10.1162/tacl_a_00232 ↩︎
-
Kirby, D. A., Chambers, A. C., & Macauley, W. R. (2015, August 10). What Entertainment Can do for Science, and Vice Versa. http://thescienceandentertainmentlab.com/what-ent-can-do-for-sci/ ↩︎
-
de Araújo, C. G. S. (2014). Detailing the Writing of Scientific Manuscripts: 25-30 Paragraphs. Arquivos Brasileiros de Cardiologia, 102(2), e21–e23. https://doi.org/10.5935/abc.20140019 ↩︎
-
Wobbrock, J. O., Hattatoglu, L., Hsu, A. K., Burger, M. A., & Magee, M. J. (2021). The Goldilocks zone: Young adults’ credibility perceptions of online news articles based on visual appearance. New Review of Hypermedia and Multimedia, 27(1–2), 51–96. https://doi.org/10.1080/13614568.2021.1889690 ↩︎
-
Brau, B. (2020). Constructivism. The Students’ Guide to Learning Design and Research. https://edtechbooks.org/studentguide/constructivism ↩︎
-
Why is the sky blue? Refraction. Why? Because science says so. Why? <- That one ↩︎
-
Sanh, V., Webson, A., Raffel, C., Bach, S. H., Sutawika, L., Alyafeai, Z., Chaffin, A., Stiegler, A., Scao, T. L., Raja, A., Dey, M., Bari, M. S., Xu, C., Thakker, U., Sharma, S. S., Szczechla, E., Kim, T., Chhablani, G., Nayak, N., … Rush, A. M. (2021). Multitask Prompted Training Enables Zero-Shot Task Generalization. ArXiv:2110.08207 [Cs]. http://arxiv.org/abs/2110.08207 ↩︎
-
Srivastava, A., Rastogi, A., Rao, A., Shoeb, A. A. M., Abid, A., Fisch, A., Brown, A. R., Santoro, A., Gupta, A., Garriga-Alonso, A., Kluska, A., Lewkowycz, A., Agarwal, A., Power, A., Ray, A., Warstadt, A., Kocurek, A. W., Safaya, A., Tazarv, A., … Wu, Z. (2022). Beyond the Imitation Game: Quantifying and extrapolating the capabilities of language models (arXiv:2206.04615). arXiv. http://arxiv.org/abs/2206.04615 ↩︎
-
Zhang, Z., Zhang, A., Li, M., Zhao, H., Karypis, G., & Smola, A. (2023). Multimodal Chain-of-Thought Reasoning in Language Models (arXiv:2302.00923). arXiv. http://arxiv.org/abs/2302.00923 ↩︎
-
agnosticmeta. (2022, December 8). Summary of War an Peace in iambic pentameter [Reddit Post]. R/ChatGPT. www.reddit.com/r/ChatGPT/comments/zg8e4z/summary_of_war_an_peace_in_iambic_pentameter/ ↩︎
-
Snyder, K. (2023, February 3). We asked ChatGPT to write performance reviews and they are wildly sexist (and racist). Fast Company. https://www.fastcompany.com/90844066/chatgpt-write-performance-reviews-sexist-and-racist ↩︎
-
Bowman, S. (2022). The Dangers of Underclaiming: Reasons for Caution When Reporting How NLP Systems Fail. Proceedings of the 60th Annual Meeting of the Association for Computational Linguistics (Volume 1: Long Papers), 7484–7499. https://doi.org/10.18653/v1/2022.acl-long.516 ↩︎
-
McDade, A. (n.d.). Studies show ChatGPT cheating is on the rise among students—Young and old—As teachers remain divided on bans. Business Insider. Retrieved 12 February 2023, from https://www.businessinsider.com/teachers-caught-students-cheating-chatgpt-survey-shows-2023-2 ↩︎
Replies & Web Activities
If you would like to comment or reply then toot me or bluesky me about this url, or send me a webmention